AI and Software Testing
With promises of increased velocity, efficiency and insight, artificial intelligence (AI) has innovators eager to incorporate AI technologies into their stack
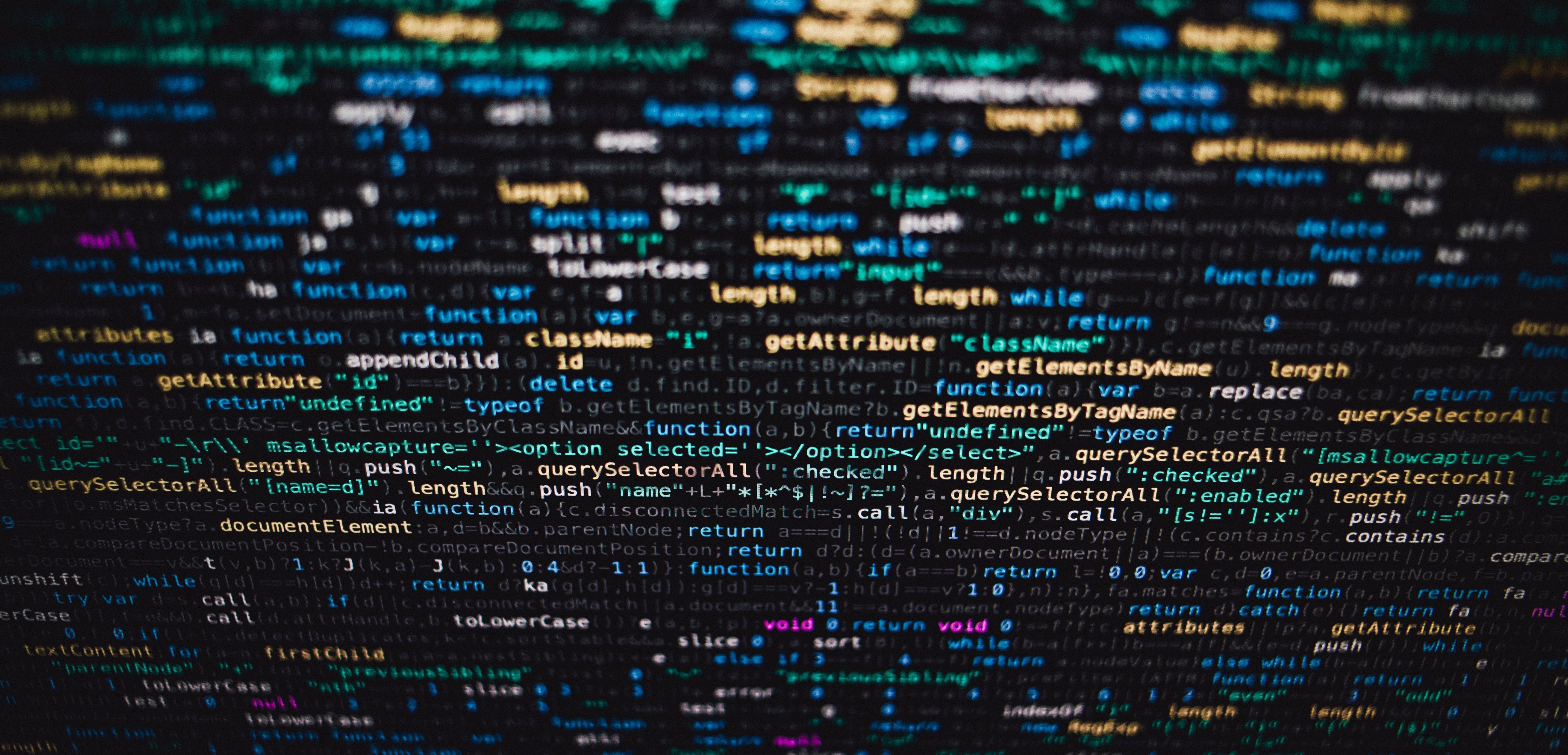
With promises of increased velocity, efficiency and insight, artificial intelligence (AI) has innovators eager to incorporate AI technologies into their stack. To stay competitive, many marketing departments have also picked up on the term, although often in this instance AI can be conflated with run-of-the-mill programming.
As a company that specializes in software testing, we wanted to explore how AI comes into play. Is it just a buzzword or are there any useful applications of AI in automated testing?
What is AI?
Before we dive into software testing with AI we should first define what we mean when we say “AI”. The most general definition of AI is that it is a synthetic machine, usually a computer program, that is capable of intelligence. By intelligence, we mean the computational ability to achieve a goal. This definition is very broad, but we can break AI down into small subcategories: Narrow and General AI. Narrow (ANI), or weak, AI is AI that has been programmed to perform a single task. Artificial General Intelligence (AGI), or Strong AI, is more human-like in that it can perform any task a person could. AGI is still in the early stages of development, so we will focus on ANI.
ANI has become fairly common; virtual assistants like the Google Assistant and Siri, Tesla vehicle’s self-driving abilities, IMB’s Watson’s healthcare solutions and even the bots that play us in chess and DOTA are all examples of ANI. As far as software testing is concerned, ANI is its best bet.
AI in software testing
I attended a conference recently where a group of leaders from the software testing world debated whether AI has become useful for testers yet. Wolfgang Platz, founder of test automation company, Tricentis, felt that we are already using AI. Though it is nowhere near AGI, Wolfgang says services like LiveCompare and the control recognition algorithms in the Tosca tool are valid applications of AI. He argues that these “smart algorithms” are just narrow applications of basic AI. As opposed to Wolfgang’s developer focused perspective, Thomas Murphy of Gartner stated that when testers or QA departments think of AI, they expect the automatic generation of test cases. “We are not there yet,” Murphy says. Outside of the ongoing research on AGIs, building a successful AI would require immense amounts of data to train it with; an effort that is compounded by a lack of standardization when it comes to building requirements and test cases. According to Platz, the cost of training an effective AI would not be worth the effort.
The future of AI in software testing
Even though we see applications of ANI all around us, its footprint in QA is still tiny. Gartner released a research paper on the many applications of AI and found that most of the expected use cases of AI lie on the “hype side” of the expectation curve.
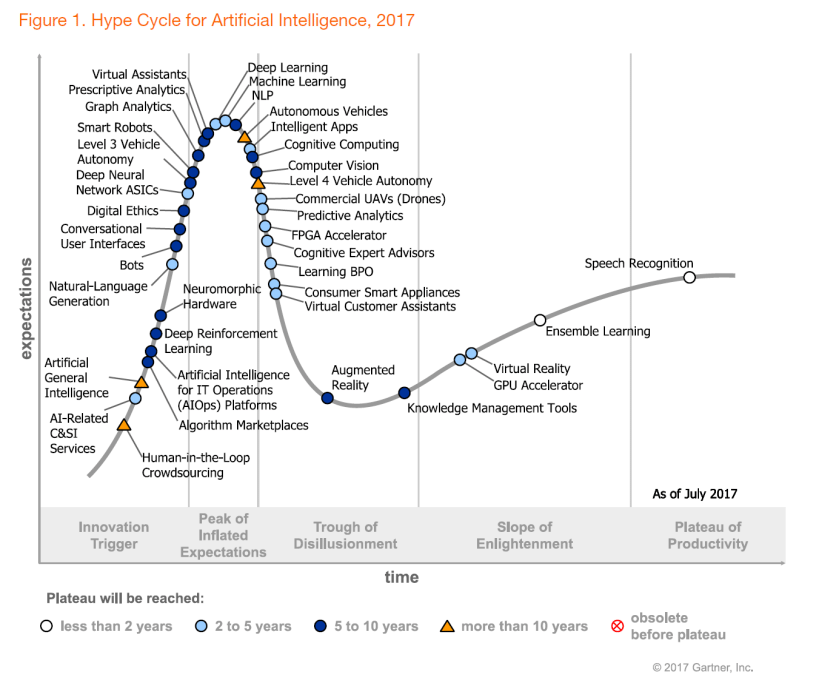
It will take more time and research before ANI or AGI can be of any real use to testers. Until then, you’d be safe in concluding that most testing tools that tout AI are probably just trying to generate buzz. Ultimately, clients are looking for ways to make QA easier or more efficient, whether it is with AI or some other technology.